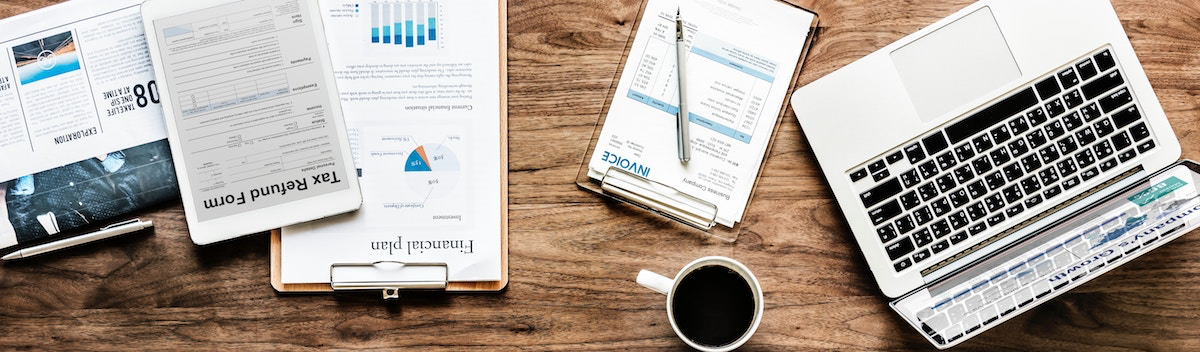
Unlock Your Valuable Data Assets
Your Valuable Asset
We all have some type of data that we collect with our companies. This data should not be viewed as a liability, but rather as an asset that can even generate meaningful value through cost reduction or cash flows. This data can actually be an incredible asset.
Even as individuals, we know that we amass extremely high levels of data and firms generate a great deal of value from this asset. Take Facebook for instance: they know a lot about us as individuals and generate staggering value by selling our attention (i.e. "eyeballs") to advertisers using the characteristics of our digital presence (i.e. our "data" footprint). We as individuals in the form of our clicks and attention are Facebook's assets. Without us, their value is massively diminished.
This is very much why early-stage companies are valued based on their page views and how companies that have a deeper understanding of their page visitors can leverage more meaningful value from this data. The leap to how data can be a significant asset for our companies is not too difficult to make looking at it through this lense.
I will give you tangible examples of how you can corral your data in such a way and utilize math and statistics (or at least give it to those who enjoy solving math and statistical problems) to increase revenue or decrease costs...or both. This affects the bottom line and makes you more money.
Where is my Asset...my Data
How do we do this with your company? We must first ask where are these assets? These assets are hopefully in our technology systems, our servers, but can also be in a filing cabinet in paper form!
If I am running a trucking company, for instance, and make my money by delivering things from Point A to Point B, then it is likely that I have trucks or other vehicles that do the heavy lifting. I also have employees who might be the most valuable asset after all (at least until my trucks begin driving themselves). These are our core assets that enable our firm to generate cash. In trucking, clients give a company cash for delivering things from A to B. The company uses people and trucks to move these things around.
I have a management background in trucking and logistics, so these trucking examples come naturally to me. I use them because they are very tangible and come naturally to most folks. Many of us order things through Amazon and are used to getting boxes dropped off on our front step by UPS.
Back to our assets...trucks and employees. These core assets have certain characteristics about them and we record these characteristics: the data. For trucks, we have fairly easy access to the basics such as when this vehicle was manufactured (i.e. its age), maintenance records, mileage logs, invoices for each delivery (the money), and who drove the vehicle. For our drivers we have access to the trips they have driven, how much they are paid, and things like vehicle accidents, etc. Many companies retain this type of data because they have to for regulatory reasons, to get paid by their customers, or to hire an employee and pay them.
This is the kind of data we need to operate successfully in this industry.
Creating Value with my Data
Now we can begin to understand how we generate value with our data. So, we are collecting the "table stakes" in terms of what we must do to run our company. Well, so is everyone else. That's the bad news. You can continue doing what you have to do to get by, but you will soon be surpassed by your competitors as they begin to understand how to leverage their data and make better decisions using it.
The first step is to begin collecting additional data or organizing your data in a way that it can be put to meaningful use. Much of the data we come in contact with we just let flitter away into digital oblivion. We do not collect and store it. We refer to this as "data exhaust" because, much like an automobile, we let those byproducts that run through our system just float away—this is the carbon and oxygen just floating away from the exhaust of an automobile. It has inherent value, but it must be restructured to provide value to someone.
Similarly, in our trucking scenario, we drive trucks from point A to B to C and so on to get to all of the stops throughout the day, but our drivers are only collecting signed invoices at each stop. This is simply table stakes in terms of what we have to be doing to run the business. We must get paid for our service. However, we can easily add a sensor to each vehicle to measure and collect the exact location, speed, acceleration and elevation over the course of the day. This opens up whole new rich dimensions of analysis that we can now access through our data.
Using this data with what we already have we can begin to answer some very valuable questions:
- What routes must my trucks drive to reduce our overall fuel cost?
- Which customers are not profitable for my business?
- What policies can I develop & implement to create more efficient driving?
- What products for certain customers should I bundle on each truck to be most efficient?
- And many more...
It's All About the Math
How do we answer these questions? What routes must my trucks drive to reduce our cost of fuel across my fleet of vehicles?
It is all about the math...and statistics. Statistics is that class that most students found incredibly boring and probably only took once because it was required for their degree. The operative word here is "most." There is a minority of students (and maybe a fairly small minority) that actually enjoyed those courses. I was one of them. I tutored other students because I enjoyed it so much. And there is an entire new profession that was born out of this field (see my other post What is a Data Scientist for more on that topic).
To solve the fuel problem and make this company's trucking operations more efficient, we utilize mathematical and statistical methods from a field called operations research. These are called "optimization" methods and are what is used to route your car in your Google Maps app on your phone.
Basically, we identify all of the possible routes (represented as segments between cities, for instance) that a truck can take and collect as much information from each as we can. We might collect the average time it takes to travel from Point A to Point B along with the grade of the road, any obstacles, height limitations from bridges, etc. This is just the beginning.
We then identify the characteristics of the jobs that we have to run. This is the most important part and would consist of all the goods that we have to get to all customers in a given day or week. We also must collect all of the characteristics of the goods such as weight and dimensions of the shipping containers and boxes. This is important because one truck probably cannot carry everything, so we also use these constraints to determine the most efficient way to pack out the vehicles. If we can keep a vehicle off the road, then that is probably the best way to save fuel.
Once we have all of that mapped out, we design and configure an algorithm to determine the optimal route. That's the magic here and this is what most Data Scientists enjoy doing the most. I will skip the gory details, but basically a bunch of super smart dudes in the 40's, 50's, 60's and 70's invented methods for doing this. This was largely driven by the massive logistical efforts of the Second Great War and was further driven by the military-industrial complex, but we reap the rewards today.
So our trucks are now using much less fuel because of math. How much?
Well, if we have 50 Isuzu NPR Box Trucks that each drive 80 miles per day on average, then they are using approximately 286 gallons per day. This costs the company ~$920 per day (at $3.217 per gallon of diesel). This costs the company $335,487 per year.
If we can reduce the fuel expenditure by 15% with our optimization model, then that is $50K ($50,323) that they can save in the first year. If they bring us in to build their optimization system, then they can pay for us to do the work in the first year or two and carry that benefit well into the future.
Everyone wins...yay!
Where do you Begin?
Where does a firm like ours get started? Great question! We start by getting to know your company and your data. We conduct an initial assessment where we interview select staff and get access to your systems so that we can understand what type of data you have and what we can do with it.
As a part of this assessment, we also build a two- to five-year roadmap for those companies that want to make more decisions in the future using data. Companies usually see their competitors making moves in this direction or stay on top of industry news that highlights these data science efforts elsewhere and deside to stick their toe in the water.
We help our customers build out these capabilities organically. This means that they will have their own staff and infrastructure doing the work in the future. This does not happen overnight, so we assist them in getting there because we have done this many times before. We identify where they are now and where they need to be and put the puzzle pieces together that will get them there successfully.
The final component of the assessment is a list of several dozen analytical opportunities that the company can start to tackle now. This effort will continue for years, but each opportunity is designed to generate a benefit now (think about that fuel savings above and dozens of additional projects in the future that build value and savings in that way).
In this way, our clients will be able to unlock the hidden value that lies within their data. They will be able to leverage their data as an asset to generate future cash flows and value.
What Now?
Contact us and let us know if your company has a desire to make better decisions using data. We don't want to replace the decision making that has made your company successful, but rather, want to enhance this decision making through data science.
Contact me directly at john@boxelderanalytics.com if you want to discuss how we can make you more profitable.
We can then talk about how our team of amazing technologists, data and math professionals can begin to usher your company into the "Data Age."